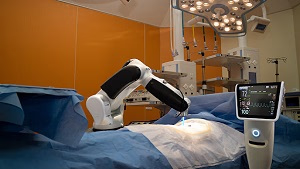
Exploring the Basics of medical Military Artificial Intelligence and its impact of traditional healthcare
Key Points: AI Potential in Healthcare
-
Intro to AI Potential in Healthcare - Revolutionizing Patient Care and Treatment, Understanding the
Impact of Artificial Intelligence on Healthcare Systems
-
AI Applications in Healthcare - Diagnostic Precision, Drug Discovery Advancements, Personalized
Medicine, Predictive Analytics, Operational Efficiency, Telehealth Innovations
-
Benefits of AI in Healthcare - Improved Patient Outcomes, Accelerated Innovation, Patient-Centred
Care, Disease Prevention
-
Ethical and Regulatory Considerations - Privacy and Security, Bias and Fairness, Regulatory
Compliance, Transparency and Accountability
-
Conclusion - Embracing the Potential of AI in Healthcare, Encouraging Continued Investment and Innovation
in AI for Healthcare Improvement, building a Sustainable and Ethical AI Healthcare Ecosystem: Key to
Realizing the Full Potential of AI in Healthcare
1. Introduction
1.1. Overview of AI in Healthcare: Revolutionizing Patient Care and Treatment
AI in healthcare is revolutionizing patient care and treatment by leveraging advanced
technologies to enhance medical diagnosis, personalized treatment plans, and overall healthcare delivery.
Here's an overview of how AI is transforming healthcare:
- Medical Imaging and Diagnosis: AI algorithms are being used to analyze medical
imaging data, such as X-rays, MRIs, and CT scans, to detect abnormalities, tumors, and other conditions
with high accuracy and efficiency. AI-powered diagnostic tools can help radiologists and clinicians make
faster and more accurate diagnoses, leading to earlier detection and treatment of diseases.
- Predictive Analytics and Early Intervention: AI-driven predictive analytics models
analyze patient data, including electronic health records (EHRs), lab results, and genetic information,
to identify patients at risk of developing certain diseases or complications. By predicting disease
progression and identifying high-risk patients, healthcare providers can intervene earlier, implement
preventive measures, and improve patient outcomes.
- Personalized Treatment Plans: AI algorithms analyze large datasets of patient
records, clinical trials, and medical research to tailor treatment plans and interventions to individual
patient characteristics, including genetic predispositions, medical history, and lifestyle factors.
Personalized medicine approaches enable healthcare providers to optimize treatment efficacy, minimize
adverse effects, and improve patient adherence to treatment regimens.
- Virtual Health Assistants and Chatbots: AI-powered virtual health assistants and
chatbots provide patients with personalized health advice, symptom assessment, and medical information
through interactive chat interfaces. These virtual assistants can triage patients, answer common health
questions, schedule appointments, and provide remote monitoring and support, enhancing access to
healthcare services and improving patient engagement and satisfaction.
- Drug Discovery and Development: AI algorithms are accelerating the drug discovery and
development process by analyzing vast amounts of biological data, genomic sequences, and chemical
compounds to identify potential drug targets, predict drug interactions, and optimize drug design.
AI-driven drug discovery platforms enable researchers to expedite the development of new therapies and
treatments for a wide range of diseases and conditions.
- Remote Patient Monitoring: AI-enabled remote patient monitoring systems use wearable
devices, sensors, and IoT technology to collect real-time health data, such as vital signs, activity
levels, and medication adherence, from patients outside of traditional healthcare settings. Remote
monitoring allows healthcare providers to track patient health status, detect early signs of
deterioration, and intervene proactively to prevent hospital readmissions and complications.
- Operational Efficiency and Resource Optimization: AI technologies streamline
healthcare operations by automating administrative tasks, optimizing resource allocation, and improving
workflow efficiency. AI-driven solutions for healthcare scheduling, staffing, inventory management, and
supply chain logistics help healthcare organizations reduce costs, improve productivity, and enhance the
quality of care delivery.
Overall, AI is revolutionizing patient care and treatment by enabling more accurate
diagnoses, personalized treatment plans, proactive interventions, and efficient healthcare delivery. By
harnessing the power of AI technologies, healthcare providers can improve patient outcomes, enhance the
patient experience, and advance the future of medicine. However, it's crucial to address challenges such as
data privacy, regulatory compliance, and ethical considerations to ensure the responsible and ethical use of
AI in healthcare.
1.2. Understanding the Impact of Artificial Intelligence on Healthcare Systems
The impact of artificial intelligence (AI) on healthcare systems is profound and
multifaceted, revolutionizing various aspects of patient care, clinical decision-making, and healthcare
delivery. Here's a comprehensive understanding of how AI is shaping healthcare systems:
- Improved Diagnostics and Disease Detection: AI-powered diagnostic algorithms analyze
medical images, such as X-rays, MRIs, and CT scans, to detect abnormalities and diseases with high
accuracy. Early detection facilitated by AI algorithms allows for timely interventions and improved
patient outcomes, especially in conditions like cancer and cardiovascular diseases.
- Personalized Treatment Plans: AI analyzes vast amounts of patient data, including
genetic information and medical histories, to develop personalized treatment plans tailored to
individual patients. Personalized medicine approaches optimize treatment efficacy, minimize adverse
effects, and improve patient adherence to treatment regimens.
- Enhanced Clinical Decision Support: AI-based clinical decision support systems
analyze patient data to provide real-time insights, recommendations, and predictions to healthcare
providers. Decision support systems help clinicians make more informed decisions, reduce diagnostic
errors, and improve patient safety and outcomes.
- Remote Patient Monitoring and Telemedicine: AI-powered remote monitoring systems use
wearable devices and sensors to collect real-time health data from patients outside of traditional
healthcare settings. Telemedicine platforms leverage AI to facilitate virtual consultations, remote
diagnosis, and remote patient management, enhancing access to healthcare services and reducing
healthcare disparities.
- Drug Discovery and Development: AI accelerates the drug discovery process by
analyzing biological data, genomic sequences, and chemical compounds to identify potential drug targets
and optimize drug design. AI-driven drug discovery platforms enable researchers to expedite the
development of new therapies and treatments for various diseases and conditions.
- Operational Efficiency and Resource Optimization: AI automates administrative tasks,
optimizes resource allocation, and improves workflow efficiency in healthcare organizations. AI-driven
solutions for scheduling, staffing, inventory management, and supply chain logistics enhance operational
efficiency, reduce costs, and improve the quality of care delivery.
- Enhanced Patient Engagement and Experience: AI-powered virtual health assistants and
chatbots provide patients with personalized health advice, symptom assessment, and medical information
through interactive chat interfaces. Virtual assistants improve patient engagement, empower patients to
manage their health proactively, and enhance the overall patient experience.
- Data Analytics and Population Health Management: AI analyzes population health data
to identify trends, patterns, and risk factors, facilitating population health management and disease
prevention initiatives. Predictive analytics models help healthcare organizations allocate resources
effectively, target interventions, and improve public health outcomes.
Overall, the impact of AI on healthcare systems is transformative, enabling more accurate
diagnoses, personalized treatment plans, proactive interventions, and efficient healthcare delivery. However,
it's essential to address challenges such as data privacy, regulatory compliance, and ethical considerations
to ensure the responsible and ethical use of AI in healthcare.
2. AI Applications in Healthcare
2.1 Diagnostic Precision: AI-powered Medical Imaging for Accurate Disease Detection
AI-powered medical imaging is revolutionizing disease detection by providing unparalleled
accuracy and efficiency in diagnostics. Here's how it works:
- Enhanced Image Analysis: AI algorithms analyze medical images, such as X-rays, MRIs,
CT scans, and mammograms, with remarkable precision. These algorithms can detect subtle patterns and
abnormalities that might be missed by human observers, leading to earlier and more accurate diagnoses.
- Improved Accuracy: By leveraging deep learning and computer vision techniques, AI
systems can accurately identify signs of diseases such as cancer, fractures, tumors, and abnormalities
in organs or tissues. This enhances the reliability of diagnostic outcomes and reduces the likelihood of
false positives or false negatives.
- Automation of Tasks: AI-powered medical imaging automates routine tasks previously
performed by radiologists and clinicians, such as image interpretation and analysis. This frees up
valuable time for healthcare professionals to focus on more complex cases and patient care, thereby
improving overall efficiency in healthcare delivery.
- Quantitative Analysis: AI algorithms provide quantitative measurements and analysis
of imaging data, allowing for precise characterization of lesions, tumor growth rates, tissue density,
and other diagnostic parameters. This quantitative data enhances clinical decision-making and enables
more personalized treatment planning for patients.
- Real-time Insights: AI-enabled medical imaging systems provide real-time insights and
feedback to healthcare providers, allowing for immediate interpretation of imaging results and timely
intervention when necessary. This enables faster diagnosis, treatment initiation, and patient
management, leading to improved outcomes and prognosis.
- Adaptive Learning: AI systems continuously learn and improve over time as they are
exposed to more data and clinical cases. This adaptive learning capability enables AI algorithms to
refine their diagnostic accuracy and performance, leading to further advancements in disease detection
and patient care.
- Accessibility and Scalability: AI-powered medical imaging solutions can be deployed
across healthcare facilities, including remote and underserved areas, improving access to diagnostic
services for patients worldwide. Additionally, these solutions offer scalability, allowing healthcare
organizations to handle large volumes of imaging data efficiently and cost-effectively.
Overall, AI-powered medical imaging holds tremendous promise for revolutionizing disease
detection and diagnosis by providing accurate, efficient, and accessible diagnostic solutions. As AI
technologies continue to evolve, they are expected to play an increasingly critical role in improving patient
outcomes, reducing healthcare costs, and advancing medical research and innovation.
2.2 Drug Discovery Advancements: Utilizing AI Algorithms to Expedite Pharmaceutical Research
Drug discovery is a complex and time-consuming process that involves identifying and
developing new therapeutic compounds to treat diseases. AI algorithms are revolutionizing this process by
accelerating the identification of promising drug candidates and streamlining the drug development pipeline.
Here's how AI is being utilized to expedite pharmaceutical research:
- Target Identification and Validation: AI algorithms analyze large biological
datasets, including genomic data, protein structures, and disease pathways, to identify potential drug
targets. By predicting the biological mechanisms underlying diseases, AI helps researchers prioritize
targets for further investigation and validation.
- Virtual Screening and Molecular Docking: AI-powered virtual screening techniques
simulate the interaction between drug candidates and target proteins, allowing researchers to identify
molecules with the greatest potential for binding and therapeutic efficacy. Molecular docking algorithms
predict the binding affinity and binding modes of small molecules to target proteins, guiding the
selection of lead compounds for experimental validation.
- Drug Design and Optimization: AI algorithms leverage machine learning and
computational modeling to design and optimize drug molecules with desired properties, such as potency,
selectivity, and pharmacokinetics. Generative models, such as generative adversarial networks (GANs) and
variational autoencoders (VAEs), generate novel chemical structures with drug-like properties, expanding
the chemical space of potential drug candidates.
- Predictive Toxicology and Safety Assessment: AI algorithms predict the potential
toxicity and safety profiles of drug candidates based on their chemical structures and biological
properties. Predictive models assess the likelihood of adverse effects, such as cardiotoxicity or
hepatotoxicity, guiding decision-making in preclinical studies and clinical trials.
- Clinical Trial Optimization: AI-powered predictive analytics optimize clinical trial
design and patient recruitment strategies to accelerate the development and approval of new drugs.
Machine learning algorithms analyze patient data and trial outcomes to identify predictive biomarkers,
stratify patient populations, and optimize dosing regimens, leading to more efficient and successful
clinical trials.
- Drug Repurposing and Combination Therapy: AI algorithms analyze large-scale
biomedical data, including drug-drug interactions, gene expression profiles, and clinical outcomes, to
identify opportunities for drug repurposing and combination therapy. By repositioning existing drugs for
new indications or identifying synergistic drug combinations, AI accelerates the translation of
preclinical research into clinical practice.
- Real-world Evidence and Post-market Surveillance: AI-driven analytics analyze
real-world data from electronic health records, patient registries, and healthcare databases to monitor
the safety and effectiveness of drugs in clinical practice. Real-world evidence informs post-market
surveillance activities, regulatory decisions, and healthcare policy-making, ensuring the continued
safety and efficacy of approved drugs.
Overall, AI algorithms are transforming pharmaceutical research and drug discovery by
expediting the identification, design, and optimization of novel therapeutics. By leveraging machine learning,
computational modeling, and big data analytics, AI accelerates the drug development pipeline, reduces costs,
and improves the success rate of bringing new drugs to market. As AI technologies continue to advance, they
hold immense promise for addressing unmet medical needs, improving patient outcomes, and advancing precision
medicine approaches in healthcare.
2.3. Personalized Medicine: Tailoring Treatment Plans Based on Individual Patient Data and
Genetic Profiles
Personalized medicine, also known as precision medicine, is a groundbreaking approach to
healthcare that involves tailoring medical treatments and interventions to the unique characteristics of
individual patients, including their genetic makeup, molecular profiles, environmental factors, and lifestyle
choices. AI algorithms play a crucial role in advancing personalized medicine by analyzing vast amounts of
patient data and genetic information to inform treatment decisions and optimize patient outcomes. Here's how
personalized medicine utilizes AI algorithms to tailor treatment plans based on individual patient data and
genetic profiles:
- Genomic Sequencing and Analysis: AI algorithms analyze genomic data obtained through
techniques such as next-generation sequencing to identify genetic variations, mutations, and biomarkers
associated with disease susceptibility, progression, and treatment response. Genomic analysis helps
clinicians identify targetable genetic alterations and molecular pathways that can inform treatment
selection and optimization for individual patients.
- Biomarker Identification and Validation: AI-powered bioinformatics tools analyze
multi-omics data, including genomics, transcriptomics, proteomics, and metabolomics, to identify
biomarkers that predict disease risk, prognosis, and treatment response. Biomarker discovery enables
clinicians to stratify patient populations, personalize treatment strategies, and monitor disease
progression or treatment efficacy over time.
- Predictive Analytics and Risk Assessment: AI-driven predictive analytics models
integrate clinical data, genetic information, and environmental factors to assess individual patient
risk for developing certain diseases or adverse health outcomes. Predictive algorithms help clinicians
identify high-risk patients who may benefit from preventive interventions, early screening, or targeted
therapies to mitigate disease progression and improve long-term outcomes.
- Treatment Optimization and Drug Selection: AI algorithms analyze patient data,
including genetic profiles, medical history, comorbidities, and treatment responses, to optimize
treatment regimens and select the most effective therapies for individual patients. Pharmacogenomic
analysis guides drug selection and dosing decisions based on genetic variations that influence drug
metabolism, efficacy, and toxicity, minimizing adverse effects and maximizing therapeutic benefits.
- Dynamic Treatment Adaptation and Monitoring: AI-driven decision support systems
continuously analyze patient data and real-time clinical information to adapt treatment plans
dynamically based on evolving patient needs, disease progression, or treatment responses. Real-time
monitoring and feedback enable clinicians to adjust treatment strategies, titrate medication doses, or
switch therapies as needed to optimize patient outcomes and minimize risks.
- Patient Stratification and Clinical Trials Design: AI algorithms stratify patient
populations into subgroups based on genetic profiles, molecular subtypes, or clinical characteristics to
identify potential responders to experimental therapies or targeted interventions. Patient
stratification informs the design of clinical trials, allowing researchers to enroll homogeneous patient
cohorts with specific genetic or molecular characteristics, increasing the likelihood of detecting
treatment effects and improving trial outcomes.
Overall, personalized medicine leverages AI algorithms to integrate genetic, clinical, and
lifestyle data to tailor treatment plans and interventions to the unique characteristics of individual
patients. By harnessing the power of AI-driven analytics, personalized medicine enables more precise
diagnosis, targeted therapies, and optimized treatment outcomes, advancing the paradigm shift towards
patient-centered healthcare and precision medicine approaches. As AI technologies continue to advance, they
hold immense promise for revolutionizing personalized medicine and improving patient outcomes across a wide
range of diseases and healthcare settings.
2.4 Predictive Analytics: Forecasting Disease Outbreaks and Identifying Health Risks with
AI-driven Insights
Predictive analytics, fueled by AI, is transforming the landscape of public health by
offering the capability to forecast disease outbreaks and pinpoint health risks with unprecedented accuracy
and speed.
By harnessing a multitude of data sources such as electronic health records, social media
trends, environmental factors, and demographic information, AI algorithms can discern subtle patterns and
correlations that might elude human observation. Through advanced machine learning techniques, these
algorithms can predict the likelihood of disease outbreaks, track the spread of infections, and anticipate
potential health crises before they escalate.
For instance, AI models can analyze historical disease data alongside real-time information
like symptom reports or travel patterns to forecast where and when outbreaks might occur. By identifying early
warning signs and high-risk populations, public health officials can implement targeted interventions such as
vaccination campaigns or targeted messaging to mitigate the impact of outbreaks.
Moreover, AI-driven insights enable proactive measures to address health risks before they
escalate into full-blown crises. By analyzing diverse datasets, AI can identify emerging health trends, assess
environmental hazards, and recognize socioeconomic factors that contribute to health disparities. This
information empowers policymakers and healthcare professionals to allocate resources more efficiently,
prioritize preventive measures, and tailor interventions to specific communities.
In essence, predictive analytics powered by AI represents a paradigm shift in public health
surveillance and response, offering the potential to save lives, reduce healthcare costs, and enhance overall
population health outcomes.
2.5 Operational Efficiency: Optimizing Healthcare Delivery and Resource Management through
AI
Technologies
Operational efficiency in healthcare delivery and resource management can be significantly
enhanced through the strategic implementation of AI technologies. Here's how AI can optimize various aspects
of healthcare operations:
- Patient Triage and Workflow Optimization: AI-powered triage systems can analyze
patient symptoms, medical history, and urgency to prioritize care delivery. By automating this process,
healthcare facilities can ensure that patients receive timely attention based on the severity of their
condition, thus reducing wait times and improving overall patient satisfaction.
- Predictive Maintenance for Medical Equipment: AI algorithms can analyze data from
medical devices to predict when maintenance or repairs are needed. By identifying potential issues
before they cause equipment failure, healthcare providers can minimize downtime, ensure equipment
reliability, and avoid disruptions in patient care.
- Supply Chain Management: AI can optimize inventory management by predicting demand
for medical supplies and medications based on historical data, current usage patterns, and external
factors such as seasonal trends or disease outbreaks. This enables healthcare facilities to maintain
adequate stock levels while minimizing excess inventory and associated costs.
- Resource Allocation and Staffing: AI-driven predictive analytics can forecast patient
admissions, emergency department visits, and other healthcare service demands. By accurately predicting
future needs, healthcare organizations can adjust staffing levels and allocate resources more
effectively, ensuring that the right personnel are available at the right time to meet patient needs.
- Clinical Decision Support Systems: AI-powered clinical decision support systems can
analyze patient data, medical literature, and best practices to assist healthcare providers in making
informed treatment decisions. By providing real-time recommendations and alerts, these systems can
improve patient outcomes, reduce medical errors, and enhance overall quality of care.
- Telemedicine and Remote Monitoring: AI-enabled telemedicine platforms and remote
monitoring devices can expand access to healthcare services and enable more efficient delivery of care,
particularly for patients in remote or underserved areas. By leveraging AI for remote diagnosis, triage,
and monitoring, healthcare providers can reach a broader patient population while reducing the need for
in-person visits.
Overall, AI technologies offer immense potential to streamline healthcare operations, improve
resource utilization, and enhance the quality and accessibility of care delivery. By embracing AI-driven
solutions, healthcare organizations can achieve greater operational efficiency, cost-effectiveness, and
ultimately, better patient outcomes.
2.6 Telehealth Innovations: Facilitating Remote Patient Monitoring and Virtual Consultations
with AI-enabled Solutions
Telehealth innovations empowered by AI are revolutionizing remote patient monitoring and
virtual consultations, ushering in a new era of accessible and personalized healthcare delivery. Here's how
AI-enabled solutions are facilitating these advancements:
- Remote Patient Monitoring (RPM): AI-powered wearable devices and sensors can
continuously collect and analyze patient health data, including vital signs, activity levels, and
medication adherence. Machine learning algorithms can identify patterns and trends in the data to detect
early signs of deterioration or health risks, enabling timely interventions and proactive management of
chronic conditions. Predictive analytics algorithms can forecast potential health complications based on
historical data and individual patient profiles, allowing healthcare providers to preemptively adjust
treatment plans and optimize patient outcomes.
- Virtual Consultations: AI-driven chatbots and virtual assistants can facilitate
initial patient assessments, symptom triage, and appointment scheduling, enhancing accessibility and
convenience for patients seeking medical advice. Natural language processing (NLP) algorithms can
analyze patient-provider interactions during virtual consultations to extract relevant information,
generate structured documentation, and assist healthcare providers in clinical decision-making. Computer
vision technologies can enable remote physical examinations by analyzing images or videos captured by
patients and providing real-time feedback to healthcare providers, improving diagnostic accuracy and
treatment planning.
- Personalized Care Plans: AI algorithms can analyze large-scale patient data,
including electronic health records, genetic information, and lifestyle factors, to generate
personalized care plans tailored to each patient's unique needs and preferences. Machine learning models
can predict individual patient responses to different treatment options, medications, or lifestyle
interventions, empowering healthcare providers to optimize treatment strategies and improve patient
outcomes. AI-driven population health management platforms can identify high-risk patient populations
and recommend targeted interventions to prevent disease progression, reduce hospitalizations, and lower
healthcare costs.
- Remote Rehabilitation and Therapy: AI-powered virtual reality (VR) and augmented
reality (AR) solutions can deliver immersive rehabilitation exercises and therapy sessions to patients
at home, enabling remote supervision by healthcare providers and promoting adherence to treatment
protocols. Motion tracking algorithms can assess patient movement and performance during virtual
rehabilitation sessions, providing real-time feedback and personalized guidance to optimize recovery and
functional outcomes.
Overall, AI-enabled telehealth solutions are reshaping the healthcare landscape by extending
the reach of medical expertise, enhancing patient engagement and empowerment, and driving improvements in
clinical outcomes and healthcare efficiency. As these technologies continue to evolve, they hold the promise
of delivering more accessible, cost-effective, and personalized healthcare for patients around the world.
3. Benefits of AI in Healthcare
3.1 Improved Patient Outcomes: Enhancing Diagnosis Accuracy and Treatment Effectiveness
Improving patient outcomes through enhanced diagnosis accuracy and treatment effectiveness is
a primary goal in healthcare, and AI technologies play a pivotal role in achieving this objective. Here's how
AI contributes to enhancing both diagnosis and treatment:
Enhanced Diagnosis Accuracy:
- Medical Imaging Analysis: AI algorithms can analyze medical images
such as X-rays, MRIs, and CT scans with remarkable accuracy, aiding radiologists and clinicians in
detecting subtle abnormalities and making accurate diagnoses.
- Pathology and Histology: AI-powered image analysis can assist
pathologists in identifying cancerous cells or tissue structures, improving the accuracy of cancer
diagnosis and guiding appropriate treatment decisions.
- Clinical Decision Support Systems (CDSS): AI-driven CDSS can analyze
patient data from electronic health records, laboratory tests, and imaging studies to provide clinicians
with evidence-based recommendations and differential diagnoses, reducing diagnostic errors and improving
patient outcomes.
Personalized Treatment Plans:
- Precision Medicine: AI algorithms can analyze genetic data,
biomarkers, and clinical characteristics to identify patient subgroups with specific treatment responses
or susceptibilities, enabling the delivery of personalized treatment plans tailored to individual
patient profiles.
- Drug Discovery and Development: AI accelerates the drug discovery
process by predicting the efficacy and safety of potential drug candidates, identifying novel drug
targets, and optimizing drug formulations, leading to the development of more effective therapies for
various diseases.
- Treatment Response Prediction: AI models can predict patient
responses
to different treatment options based on clinical data and historical treatment outcomes, enabling
clinicians to select the most appropriate and effective therapies for each patient while minimizing
adverse effects and treatment failures.
Remote Monitoring and Follow-up:
-
- Remote Patient Monitoring (RPM): AI-powered RPM solutions enable
continuous monitoring of patient health parameters, facilitating early detection of complications or
deterioration and enabling timely interventions to prevent adverse outcomes.
- Telehealth and Virtual Care: AI-enabled telehealth platforms
facilitate remote consultations, follow-up visits, and medication management, improving access to care
and patient compliance while reducing the risk of hospital readmissions and unnecessary healthcare
utilization.
Clinical Trials and Research:
-
- Patient Stratification: AI algorithms can analyze diverse datasets to
identify patient subgroups for clinical trials based on genetic, demographic, and clinical
characteristics, facilitating the recruitment of eligible participants and improving the likelihood of
trial success.
- Real-world Evidence Analysis: AI enables the analysis of real-world
patient data from electronic health records, claims databases, and wearable devices to generate insights
into treatment effectiveness, safety, and long-term outcomes, informing clinical practice and healthcare
policymaking.
- By harnessing the power of AI, healthcare providers can enhance diagnosis accuracy,
personalize treatment approaches, improve patient monitoring and follow-up, and drive advancements in
clinical research, ultimately leading to better patient outcomes and improved quality of care.
3.2 Accelerated Innovation: Shortening Drug Development Cycles and Expanding Treatment
Options
Accelerating innovation in drug development and expanding treatment options are critical
endeavors in healthcare, and AI technologies are playing a transformative role in achieving these goals.
Here's how AI facilitates the acceleration of drug development cycles and the expansion of treatment options:
Target Identification and Drug Discovery:
- AI-Driven Target Identification: Machine learning algorithms analyze vast biological
datasets to identify novel drug targets and pathways implicated in disease pathogenesis, accelerating
the early stages of drug discovery.
- Virtual Screening and Molecular Design: AI models predict the binding affinity and
pharmacological properties of potential drug candidates, enabling virtual screening of compound
libraries and the design of optimized drug molecules with enhanced efficacy and safety profiles.
- Generative Chemistry: AI-driven generative models can propose novel chemical
structures with desired pharmacological properties, expediting the exploration of chemical space and the
discovery of lead compounds for further development.
Predictive Toxicology and Safety Assessment:
- In Silico Toxicology: AI algorithms predict the toxicity and safety profiles of drug
candidates based on molecular structures and biological data, facilitating early identification of
potential safety issues and guiding decision-making in lead optimization and candidate selection.
- Adverse Event Prediction: AI models analyze real-world patient data and post-market
surveillance data to predict adverse drug reactions and safety risks associated with marketed
medications, informing risk management strategies and regulatory decisions.
Clinical Trial Optimization:
- Patient Selection and Recruitment: AI algorithms analyze electronic health records,
genomic data, and clinical trial databases to identify eligible patients and predict patient responses
to
investigational treatments, enhancing patient stratification and recruitment in clinical trials.
- Trial Design Optimization: AI-powered trial design platforms optimize study
protocols,
sample sizes, and endpoints to maximize statistical power and efficiency, reducing trial costs and
timelines while ensuring robust evidence generation.
- Real-time Monitoring and Data Analysis: AI enables real-time monitoring of clinical
trial data, facilitating adaptive trial designs, interim analyses, and decision-making based on emerging
trends and patient outcomes, accelerating the pace of clinical development.
Precision Medicine and Personalized Therapies:
- Biomarker Discovery and Patient Stratification: AI identifies predictive biomarkers
and
patient subgroups for targeted therapies, enabling the development of precision medicine approaches
tailored to individual patient profiles and disease characteristics.
- Drug Repurposing and Combination Therapies: AI-driven drug repurposing algorithms
identify new therapeutic uses for existing medications and predict synergistic drug combinations,
expanding treatment options and accelerating clinical translation.
- By harnessing AI-driven approaches in drug discovery, development, and clinical research,
pharmaceutical
companies and research institutions can accelerate innovation, shorten drug development cycles, and
bring
novel treatments to patients more rapidly, ultimately improving health outcomes and addressing unmet
medical needs.
3.3 Patient-Centered Care: Empowering Individuals with Personalized Healthcare Plans and
Remote Monitoring
Patient-centered care, empowered by personalized healthcare plans and remote monitoring,
places individuals
at
the forefront of their own health journey. Here's how personalized healthcare plans and remote monitoring
technologies empower individuals:
Personalized Healthcare Plans:
- Tailored Treatment Strategies: Individuals receive personalized treatment plans based
on
their unique medical history, genetic makeup, lifestyle factors, and preferences. These plans consider
the
individual's specific health goals and values, leading to more effective and patient-centric care.
- Shared Decision-Making: Patients are actively involved in decision-making processes,
collaborating with healthcare providers to choose treatment options that align with their preferences,
values, and circumstances. This shared decision-making approach enhances patient autonomy and
satisfaction
while improving treatment adherence and outcomes.
- Continuous Optimization: Healthcare plans are continuously optimized based on
real-time
health data, patient feedback, and evolving health needs. This iterative process ensures that care
remains
personalized and responsive to changes in the individual's health status and goals over time.
Remote Monitoring:
- Continuous Health Monitoring: Individuals benefit from continuous monitoring of their
health parameters, including vital signs, symptoms, medication adherence, and activity levels, using
wearable devices, mobile apps, and remote monitoring platforms.
- Early Detection of Health Changes: Remote monitoring technologies enable the early
detection of changes in health status or disease progression, allowing for timely interventions and
preventive measures to prevent complications and improve outcomes.
- Patient Engagement and Empowerment: Individuals are empowered to take an active role
in
managing their health by monitoring their own health data, setting personalized health goals, and
receiving timely feedback and support from healthcare providers. This increased engagement fosters a
sense
of ownership and accountability for one's health and well-being.
Health Education and Self-Management:
- Access to Information: Individuals have access to educational resources, digital
tools,
and self-management programs that empower them to make informed decisions about their health and
lifestyle
choices. This access to information promotes health literacy and enables individuals to proactively
manage
their health and prevent disease.
- Behavioral Support and Coaching: Remote monitoring platforms may offer behavioral
support and coaching services to help individuals adopt healthy behaviors, adhere to treatment plans,
and
achieve their health goals. These interventions may include personalized feedback, goal setting, and
motivational strategies tailored to the individual's needs and preferences.
Coordination of Care:
- Interdisciplinary Collaboration: Healthcare providers collaborate across disciplines
and
settings to deliver integrated, patient-centered care that addresses the individual's holistic health
needs. This coordination ensures continuity of care, minimizes fragmentation, and optimizes health
outcomes.
- Care Coordination Platforms: Digital platforms facilitate communication and
information
sharing among members of the healthcare team, as well as between healthcare providers and patients.
These
platforms streamline care coordination, enhance communication, and improve the patient experience.
- By leveraging personalized healthcare plans and remote monitoring technologies, individuals are
empowered
to actively participate in their own care, make informed decisions, and achieve better health outcomes.
This patient-centered approach fosters a collaborative partnership between individuals and healthcare
providers, ultimately leading to improved quality of care and patient satisfaction.
3.4 Disease Prevention: Proactively Identifying Risk Factors and Implementing Preventive
Measures
Proactive disease prevention involves identifying risk factors early and implementing
preventive measures to
reduce the likelihood of disease occurrence or progression. Here's how healthcare strategies can achieve this:
Risk Factor Identification:
- Data Analysis and Prediction: Analyzing population health data, genetic information,
lifestyle factors, and environmental exposures can help identify individuals at higher risk for certain
diseases. AI and machine learning algorithms can analyze large datasets to detect patterns and
correlations, allowing for the early identification of risk factors.
- Genetic Screening: Genetic testing can identify individuals with genetic
predispositions
to certain diseases, enabling targeted interventions and personalized preventive measures.
- Health Risk Assessments: Conducting health risk assessments and screenings can
identify
modifiable risk factors such as high blood pressure, obesity, smoking, and sedentary lifestyle, which
contribute to the development of chronic diseases.
Preventive Measures:
- Health Promotion and Education: Providing health education and promoting healthy
lifestyle behaviors such as regular exercise, balanced nutrition, smoking cessation, and stress
management
can reduce the incidence of chronic diseases and improve overall health outcomes.
- Vaccination Programs: Implementing vaccination programs against infectious diseases
can
prevent outbreaks and reduce disease transmission within communities, contributing to population-level
disease prevention.
- Screening and Early Detection: Implementing screening programs for common diseases
such
as cancer, cardiovascular disease, and diabetes can facilitate early detection and treatment, improving
prognosis and reducing disease-related morbidity and mortality.
- Behavioral Interventions: Offering behavioral interventions such as counseling,
coaching, and support groups can help individuals modify unhealthy behaviors and adhere to preventive
measures, leading to better health outcomes.
- Population Health Management: Employing population health management strategies,
including targeted interventions and outreach efforts, can address health disparities and improve access
to preventive care, particularly among underserved populations.
Technology-enabled Solutions:
- Remote Monitoring and Telehealth: Leveraging remote monitoring technologies and
telehealth platforms can facilitate proactive health monitoring, enable early detection of health
changes,
and provide timely interventions, especially for individuals with chronic conditions or high-risk
factors.
- Mobile Health Apps: Mobile health applications can promote self-monitoring of health
behaviors, track progress towards health goals, and deliver personalized health recommendations and
interventions, empowering individuals to take control of their health.
- Predictive Analytics: Utilizing predictive analytics to forecast disease trends,
identify high-risk populations, and allocate resources strategically can enhance the effectiveness of
preventive interventions and optimize population health outcomes.
- By proactively identifying risk factors and implementing preventive measures, healthcare systems can
reduce the burden of disease, improve population health, and mitigate the economic and social impact of
preventable illnesses. This preventive approach emphasizes the importance of early intervention, health
promotion, and empowering individuals to make healthy choices for themselves and their communities.
3.5 Streamlined Operations: Reducing Administrative Burden and Enhancing Workflow Efficiency
for Healthcare
Providers
Streamlining operations to reduce administrative burden and enhance workflow efficiency is
crucial for
healthcare providers to focus more on patient care. Here's how it can be achieved:
Automation of Administrative Tasks:
- Electronic Health Records (EHR) Optimization: Implementing user-friendly EHR systems
with intuitive interfaces and customizable templates can streamline documentation processes, reduce data
entry errors, and improve information accessibility for healthcare providers.
- Automated Appointment Scheduling: Introducing online appointment scheduling systems
and
automated reminders can streamline the appointment booking process, reduce no-show rates, and minimize
administrative workload for staff.
- Billing and Coding Automation: Utilizing automated billing and coding software can
simplify revenue cycle management, accelerate claims processing, and ensure accurate reimbursement for
services rendered.
- Insurance Verification: Implementing automated insurance verification processes can
streamline eligibility checks, reduce denials, and minimize administrative delays in obtaining prior
authorizations for procedures and treatments.
Digital Communication and Collaboration Tools:
- Secure Messaging Platforms: Implementing secure messaging platforms for intra-team
communication and collaboration can facilitate real-time communication, streamline care coordination,
and
reduce reliance on inefficient communication methods such as phone calls and faxes.
- Telemedicine Platforms: Integrating telemedicine platforms into practice workflows
can
enable virtual consultations, follow-up visits, and remote monitoring, reducing the need for in-person
appointments and enhancing patient access to care.
- Virtual Meeting Solutions: Leveraging virtual meeting solutions for team meetings,
case
discussions, and continuing education sessions can minimize the need for physical gatherings, save time,
and accommodate flexible scheduling for healthcare providers.
Standardization and Protocol Implementation:
- Clinical Pathways and Protocols: Developing standardized clinical pathways and
protocols
for common procedures, treatments, and conditions can promote consistency in care delivery, reduce
variation in practice patterns, and optimize resource utilization.
- Order Sets and Templates: Creating order sets and templates for common orders,
prescriptions, and procedures within EHR systems can streamline documentation, reduce decision fatigue,
and ensure adherence to evidence-based practices.
- Quality Improvement Initiatives: Implementing quality improvement initiatives and
performance metrics can identify areas for workflow optimization, measure progress over time, and drive
continuous process improvement efforts.
Training and Support:
- Provider Training Programs: Offering training programs and continuing education
opportunities for healthcare providers on efficient documentation practices, use of technology tools,
and
workflow optimization strategies can enhance proficiency and confidence in using digital solutions.
- Technical Support Resources: Providing readily accessible technical support
resources,
including help desks, online tutorials, and user manuals, can address user queries and troubleshooting
issues promptly, minimizing disruptions to workflow.
- By implementing these strategies, healthcare providers can streamline operations, reduce
administrative
burden, and enhance workflow efficiency, allowing them to focus more on delivering high-quality patient
care and improving patient outcomes.
3.6 Accessibility and Affordability: Increasing Healthcare Access and Reducing Costs through
Telemedicine and
AI-driven Solutions
Increasing healthcare access and reducing costs are critical goals in improving healthcare
delivery, and
telemedicine and AI-driven solutions play pivotal roles in achieving these objectives:
Telemedicine for Remote Consultations:
- Geographic Accessibility: Telemedicine enables individuals in rural or underserved
areas
to access healthcare services remotely, overcoming barriers related to distance and travel.
- Convenient Access: Telemedicine allows patients to consult with healthcare providers
from the comfort of their homes, eliminating the need for transportation and reducing time away from
work
or other commitments.
Specialist Consultations: Telemedicine facilitates access to specialist care, enabling
patients to receive expert consultations without the need for referrals or lengthy wait times.
AI-driven Triage and Decision Support:
- Efficient Triage: AI-powered chatbots and virtual assistants can triage patient
symptoms, provide initial assessments, and direct individuals to appropriate levels of care, reducing
unnecessary emergency department visits and optimizing resource utilization.
- Clinical Decision Support: AI algorithms analyze patient data and medical literature
to
provide clinicians with evidence-based recommendations, aiding in diagnosis, treatment planning, and
medication management, thereby improving efficiency and reducing medical errors.
Remote Monitoring and Chronic Disease Management:
- Continuous Monitoring: Remote monitoring technologies allow healthcare providers to
monitor patients' vital signs, symptoms, and medication adherence remotely, enabling early detection of
health changes and timely interventions, particularly for individuals with chronic conditions.
- Preventive Care: AI-driven predictive analytics can identify individuals at high risk
for adverse health outcomes and target preventive interventions to mitigate risks, reducing
hospitalizations and emergency room visits.
Cost Reduction and Efficiency Improvements:
- Reduced Healthcare Costs: Telemedicine reduces healthcare costs associated with
transportation, hospital readmissions, and unnecessary office visits, making healthcare more affordable
and accessible for patients.
- Operational Efficiency: AI-driven solutions streamline administrative processes,
automate routine tasks, and optimize resource allocation, reducing overhead costs and improving workflow
efficiency for healthcare organizations.
Health Education and Self-management:
- Patient Empowerment: Telemedicine platforms and AI-driven health apps provide
patients
with access to educational resources, self-management tools, and personalized health coaching,
empowering
individuals to take control of their health and make informed decisions.
- Preventive Measures: Health education and behavior change interventions delivered
through telemedicine and AI-driven platforms promote healthy behaviors, early detection of diseases, and
proactive management of chronic conditions, ultimately reducing healthcare costs associated with
preventable illnesses.
- By leveraging telemedicine and AI-driven solutions, healthcare providers can expand access to care,
reduce healthcare costs, improve health outcomes, and enhance the overall patient experience, ultimately
advancing the goal of accessible and affordable healthcare for all.
4. Ethical and Regulatory Considerations
Ethical and regulatory considerations are paramount in the development and deployment of
AI-driven solutions in healthcare. Here's how each aspect can be addressed:
4.1 Privacy and Security:
- Data Encryption and Access Controls: Implement robust encryption
methods and
access controls to safeguard patient data from unauthorized access or breaches.
- Compliance with Regulations: Ensure compliance with privacy
regulations such
as HIPAA (Health Insurance Portability and Accountability Act) in the United States or GDPR (General
Data
Protection Regulation) in the European Union to protect patient privacy and confidentiality.
- Data Minimization: Minimize the collection and retention of
unnecessary
patient data to reduce the risk of privacy breaches and unauthorized access.
4.2 Bias and Fairness:
- Bias Detection and Mitigation: Regularly audit AI algorithms for
biases and
disparities in data sources, ensuring fairness and equity in decision-making processes.
- Diverse Training Data: Incorporate diverse and representative
datasets
during
AI model development to mitigate biases and ensure equitable outcomes across different demographic
groups.
- Transparency in Algorithms: Provide transparency into the
decision-making
process of AI algorithms, including how data is collected, analyzed, and used to make predictions or
recommendations.
4.3 Regulatory Compliance:
- Regulatory Alignment: Ensure that AI-driven healthcare
solutions adhere to
relevant regulations and standards, including FDA (Food and Drug Administration) approvals for
medical devices
and compliance with international standards for data privacy and security.
- Ethical Review Boards: Establish ethical review boards or
committees to
oversee the development and deployment of AI systems in healthcare settings, ensuring adherence to
ethical
principles and regulatory requirements.
4.4 Transparency and Accountability:
-
- Clear Documentation: Provide clear documentation of AI
algorithms, including
their capabilities, limitations, and potential biases, to promote transparency and facilitate
informed
decision-making by healthcare providers and patients.
- Oversight and Governance: Establish governance structures and
processes to
oversee the deployment and usage of AI systems in healthcare, including mechanisms for
accountability and
recourse in case of errors or adverse outcomes.
- By addressing these ethical and regulatory considerations, healthcare
organizations can
ensure
the responsible development and deployment of AI-driven solutions, safeguard patient privacy and
security,
promote fairness and equity in decision-making, and maintain compliance with relevant regulations
and
standards.
This approach fosters trust and confidence in AI technologies among healthcare providers,
patients, and
regulatory authorities, ultimately advancing the goals of improved healthcare delivery and patient
outcomes.
5. Conclusion
Ultimately, the future of healthcare lies in our ability to harness the power of AI
responsibly
and ethically. By working together to overcome challenges and seize opportunities, we can realize the
full
potential of AI to transform patient care and shape a healthier, more equitable world for generations to
come.
5.1 Embracing the Potential of AI in Healthcare: Transforming the Future of Patient
Care and
Treatment
The integration of AI technologies into healthcare holds immense promise for
revolutionizing
patient care and treatment. From diagnosis to treatment optimization and beyond, AI has the potential to
enhance
efficiency, accuracy, and accessibility across the entire healthcare continuum.
By harnessing the power of AI-driven solutions, healthcare providers can unlock new
insights,
streamline operations, and deliver personalized care tailored to individual patient needs and
preferences.
This
transformative approach not only improves clinical outcomes but also enhances the overall patient
experience.
5.2 Encouraging Continued Investment and Innovation in AI for Healthcare Improvement
It is imperative to continue investing in research, development, and implementation of
AI
technologies in healthcare. By fostering collaboration between healthcare stakeholders, technology
developers,
and regulatory bodies, we can accelerate the adoption of AI-driven solutions and realize their full
potential
in
driving healthcare improvement.Policymakers, healthcare leaders, and industry stakeholders must prioritize
funding and
support
for AI initiatives, ensuring that innovative technologies are accessible and affordable for all
healthcare
settings and patient populations.
5.3 Building a Sustainable and Ethical AI Healthcare Ecosystem: Key to Realizing the
Full
Potential of AI in Healthcare
As we embrace the transformative power of AI in healthcare, it is essential to
prioritize
ethical considerations and regulatory compliance to build a sustainable and responsible AI healthcare
ecosystem.
By promoting transparency, fairness, privacy, and accountability in the development and
deployment of AI-driven solutions, we can mitigate risks, build trust, and maximize the benefits of AI
technologies for patients, healthcare providers, and society as a whole.In conclusion, AI has the potential to
revolutionize healthcare delivery, improve
patient
outcomes, and transform the future of medicine. By embracing innovation, fostering collaboration, and
upholding
ethical standards, we can harness the full potential of AI to create a more efficient, accessible, and
equitable
healthcare system for all. Let us continue to innovate, invest, and work together to shape a brighter
future
for
healthcare through the power of AI.